Privacy Preserving Machine Learning
Protect sensitive data while harnessing the power of machine learning with WDAI's Privacy Preserving Machine Learning service, where advanced techniques ensure data security and privacy while enabling accurate insights and predictions.
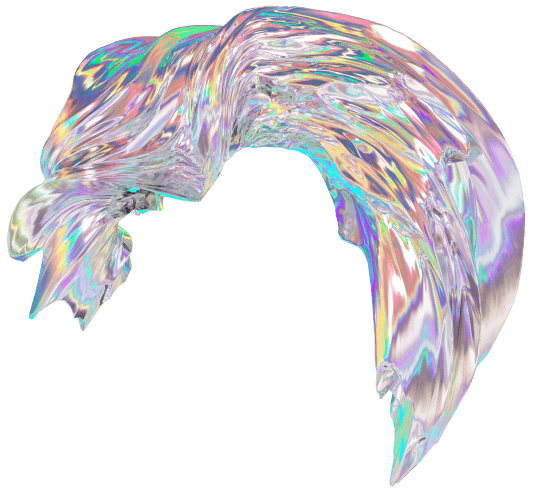
WDAI's Privacy Preserving Machine Learning service is your trusted partner in leveraging the potential of machine learning while upholding the utmost data privacy and security standards. In an era of heightened privacy concerns and strict regulatory requirements, organizations must navigate the fine balance between innovation and protecting sensitive information. Our service is designed to address this critical need, working closely with your team to understand your data, privacy requirements, and machine learning goals. Our skilled data scientists and engineers leverage state-of-the-art privacy-preserving techniques, including federated learning, secure multiparty computation, and homomorphic encryption, to develop and implement machine learning models that operate directly on encrypted data or ensure that data remains on your premises. Whether it's healthcare, finance, or any data-sensitive field, our approach empowers your organization to harness the insights of machine learning while maintaining data privacy and compliance. With WDAI's Privacy Preserving Machine Learning service, you unlock the potential of machine learning without compromising the privacy and security of your valuable data assets.
Our Privacy Preserving Machine Learning service encompasses a comprehensive range of features designed to safeguard your data while enabling accurate and insightful machine learning outcomes. We specialize in federated learning, allowing multiple parties to collaborate on model training without sharing raw data. Our service includes secure multiparty computation, enabling computations on encrypted data without revealing the actual information. We also focus on homomorphic encryption, allowing data to remain encrypted even during processing, ensuring privacy at every stage. Furthermore, we emphasize on-device machine learning, enabling AI models to operate directly on user devices, minimizing data transfers. Additionally, our experts develop data anonymization techniques, ensuring that personally identifiable information (PII) is protected while maintaining dataset utility. We also offer differential privacy, adding noise to data to protect individual privacy while maintaining data integrity. With WDAI's Privacy Preserving Machine Learning service, you embrace a new era of responsible and privacy-conscious machine learning, enabling your organization to innovate with confidence and integrity.
Federated Learning for Collaborative Insights
- Collaboration to comprehend your data, privacy requirements, and machine learning goals
- Implementation of federated learning techniques for collaborative model training without raw data sharing
- Utilization of distributed learning protocols to aggregate insights without compromising data privacy
- Integration of secure data sharing mechanisms that empower organizations to pool resources and knowledge
Secure Multiparty Computation
- Design and implementation of secure multiparty computation mechanisms for privacy-preserving computations
- Utilization of encryption and cryptographic techniques to perform computations without exposing raw data
- Development of secure protocols that enable parties to collaborate on data analysis while preserving privacy
- Creation of solutions that ensure data remains encrypted and confidential throughout computations
Homomorphic Encryption for Data Privacy
- Integration of homomorphic encryption techniques to enable data processing on encrypted data
- Utilization of encryption schemes that allow data to remain encrypted even during computation
- Development of solutions that ensure privacy at every stage of machine learning and data analysis
- Creation of systems that empower organizations to derive insights without exposing sensitive information
On-Device Machine Learning for Local Processing
- Implementation of on-device machine learning techniques for processing data directly on user devices
- Utilization of edge computing to minimize data transfers and enhance data privacy
- Development of AI models that operate locally, reducing the need for centralized data storage
- Creation of solutions that empower users to leverage machine learning while maintaining control over their data
Data Anonymization for PII Protection
- Design and development of data anonymization techniques to protect personally identifiable information (PII)
- Utilization of masking and perturbation techniques to obfuscate sensitive data while preserving utility
- Development of solutions that enable data analysis while ensuring individual privacy is maintained
- Integration of anonymization protocols that comply with data privacy regulations and standards
Differential Privacy for Privacy-Preserving Insights
- Integration of differential privacy techniques to add noise to data and protect individual privacy
- Utilization of algorithms that provide statistical guarantees of privacy while maintaining data integrity
- Development of solutions that enable organizations to extract insights without revealing sensitive information
- Creation of systems that ensure responsible and privacy-conscious data analysis and machine learning
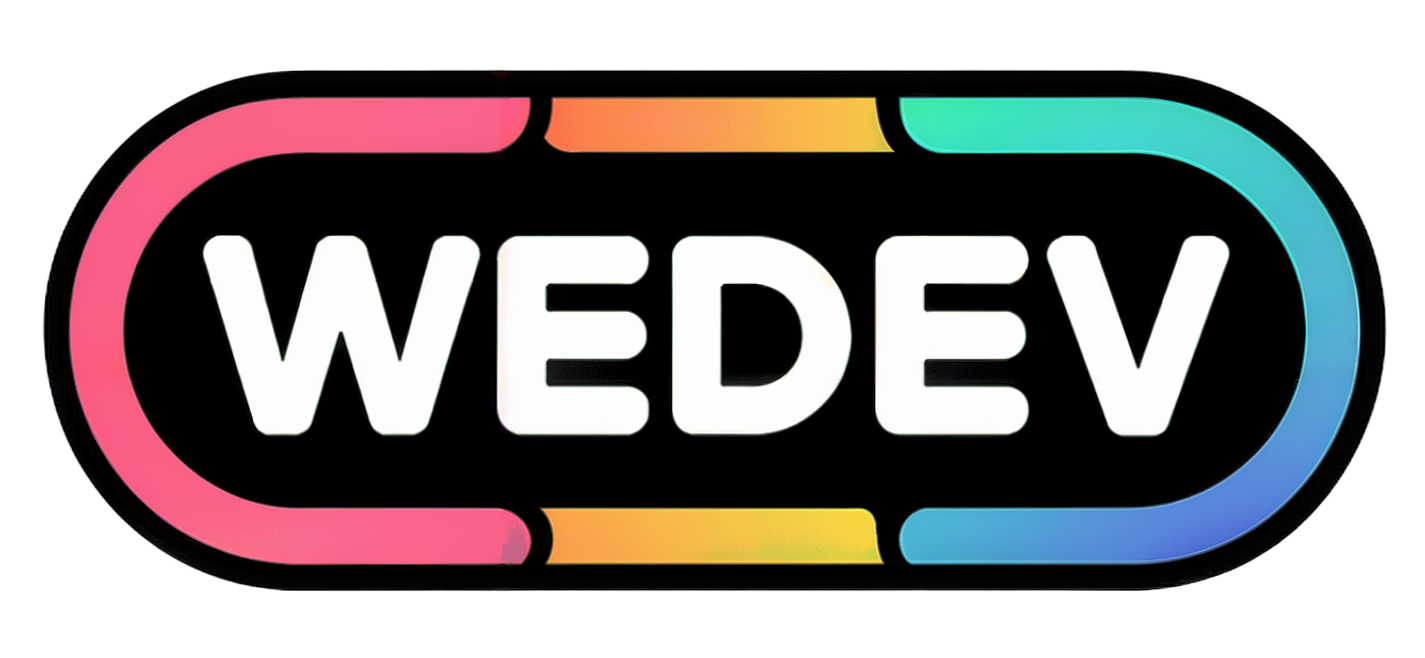